正文
博客地址:https://www.cnblogs.com/zylyehuo/
开发环境
- anaconda
- 集成环境:集成好了数据分析和机器学习中所需要的全部环境
- 安装目录不可以有中文和特殊符号
- jupyter
- anaconda提供的一个基于浏览器的可视化开发工具
import pandas as pd
import numpy as np
级联操作 -- 对应表格
- pd.concat
- pd.append
- pandas使用pd.concat函数,与np.concatenate函数类似,只是多了一些参数:
- objs
- axis=0
- keys
- join='outer' / 'inner':表示的是级联的方式,outer会将所有的项进行级联(忽略匹配和不匹配),而inner只会将匹配的项级联到一起,不匹配的不级联
- ignore_index=False
匹配级联
df1 = pd.DataFrame(data=np.random.randint(0,100,size=(5,3)),columns=['A','B','C'])
df2 = pd.DataFrame(data=np.random.randint(0,100,size=(5,3)),columns=['A','D','C'])
pd.concat((df1,df2),axis=1) # 行列索引都一致的级联叫做匹配级联
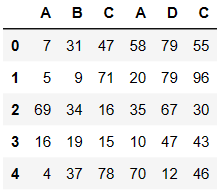
不匹配级联
- 不匹配指的是级联的维度的索引不一致。例如纵向级联时列索引不一致,横向级联时行索引不一致
- 有2种连接方式:
- 外连接:补NaN(默认模式)
- 内连接:只连接匹配的项
pd.concat((df1,df2),axis=0)
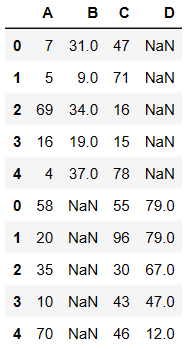
内连接
pd.concat((df1,df2),axis=0,join='inner') # inner直把可以级联的级联不能级联不处理
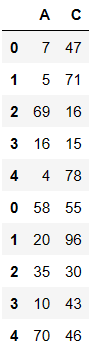
外连接
- 如果想要保留数据的完整性必须使用 outer(外连接)
pd.concat((df1,df2),axis=0,join='outer')
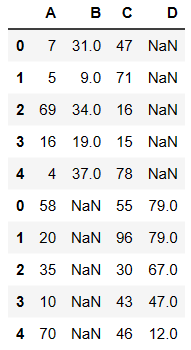
df1.append(df2)
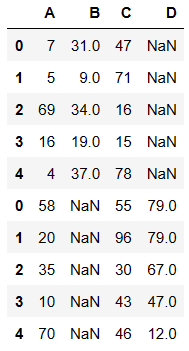
合并操作 -- 对应数据
- merge与concat的区别在于,merge需要依据某一共同列来进行合并
- 使用pd.merge()合并时,会自动根据两者相同column名称的那一列,作为key来进行合并。
- 注意每一列元素的顺序不要求一致
一对一合并
from pandas import DataFrame
df1 = DataFrame({'employee':['Bob','Jake','Lisa'],
'group':['Accounting','Engineering','Engineering'],
})
df1
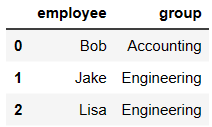
df2 = DataFrame({'employee':['Lisa','Bob','Jake'],
'hire_date':[2004,2008,2012],
})
df2
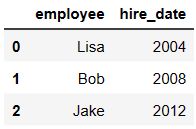
pd.merge(df1,df2,on='employee')
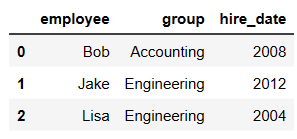
一对多合并
df3 = DataFrame({
'employee':['Lisa','Jake'],
'group':['Accounting','Engineering'],
'hire_date':[2004,2016]})
df3
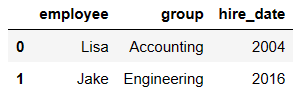
df4 = DataFrame({'group':['Accounting','Engineering','Engineering'],
'supervisor':['Carly','Guido','Steve']
})
df4
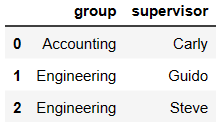
pd.merge(df3,df4) # on如果不写,默认情况下使用两表中公有的列作为合并条件
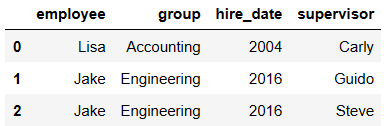
多对多合并
df1 = DataFrame({'employee':['Bob','Jake','Lisa'],
'group':['Accounting','Engineering','Engineering']})
df1
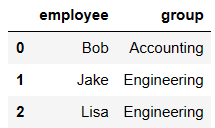
df5 = DataFrame({'group':['Engineering','Engineering','HR'],
'supervisor':['Carly','Guido','Steve']
})
df5
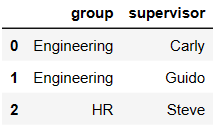
pd.merge(df1,df5,how='right')
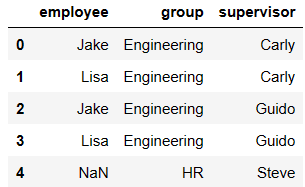
pd.merge(df1,df5,how='left')
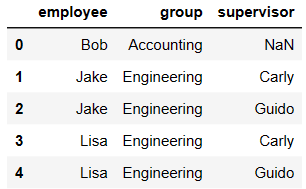
key的规范化
- 当两张表没有可进行连接的列时,可使用left_on和right_on手动指定merge中左右两边的哪一列列作为连接的列
df1 = DataFrame({'employee':['Bobs','Linda','Bill'],
'group':['Accounting','Product','Marketing'],
'hire_date':[1998,2017,2018]})
df1
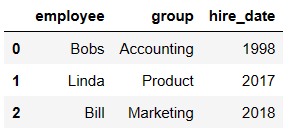
df5 = DataFrame({'name':['Lisa','Bobs','Bill'],
'hire_dates':[1998,2016,2007]})
df5
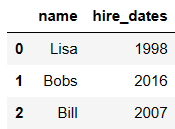
pd.merge(df1,df5,left_on='employee',right_on='name')
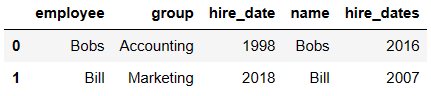
内合并与外合并
df6 = DataFrame({'name':['Peter','Paul','Mary'],
'food':['fish','beans','bread']}
)
df7 = DataFrame({'name':['Mary','Joseph'],
'drink':['wine','beer']})
df6
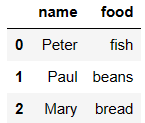
df7
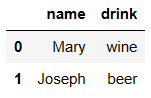
pd.merge(df6,df7,how='outer')
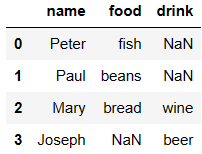
df6 = DataFrame({'name':['Peter','Paul','Mary'],
'food':['fish','beans','bread']}
)
df7 = DataFrame({'name':['Mary','Joseph'],
'drink':['wine','beer']})
df6
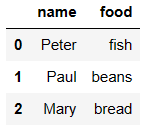
df7
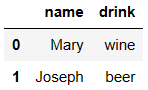
pd.merge(df6,df7,how='inner')
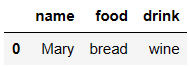